How to manage risk with business intelligence
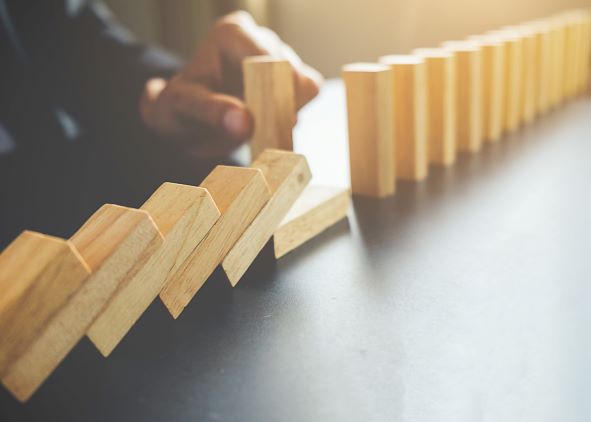
Risk: It’s the one thing that everyone wants to avoid in life, or at least manage.
No matter the industry, the risks in life are factors that must be dealt with.
In a 1686 London coffeehouse, a business of quantifying risk was created, and it shaped the British Empire and the rest of the economies all based on data. The business assessed the risks associated with shipping ventures in times of peace and in times of war.
This is the story of Llyod’s of London. The only thing that has changed since then is the speed with which data can be gathered and the number of data points that can be assessed. What hasn’t changed is the appetite to mitigate, control or otherwise manage risk factors that would limit productivity, performance, optimization and efficiency.
The epiphany for me that started my data analytics company was when I saw that no matter the business or industry, seemingly everything came down to the ability to manage data. Banking, pharmaceutical, healthcare — it all came down to having the data in a place and in a form to extract insights. It became apparent that if I could manage data more effectively, I could manage risk, efficiency or whatever came my way.
What’s always been exciting to me is how some of the questions that would arise could be solved by data modeling, visualization or analytics. For example, I remember one day when my wife came home from a hard day at work, and she was frustrated by the number of patients who were injured due to falls across a system of facilities at which she worked. The falls were being logged and duly noted, but they could not find a way to reduce the falls. She asked if there was a way to improve patient care and manage the risk of patients being injured due to falls. And by looking at the right data, I found that there was.
Many of the projects my company works on, though different from one another, have one thing in common: managing data and extracting insights.
Best Practices For Data Warehousing And Design
When leveraging data and extracting insights yourself, the challenge is first getting control of the data. Then, as far as best practices go, there a few things to be aware of:
First, to be usable, data must conform to a set of standards and be structured so that extraction for modeling, analytics and forecasting can occur to get the insights from the data. These standards incorporate a process of validation to ensure the data is clean and reliable to provide accurate analytics. Additionally, these standards are prescribed by your own organization to ensure your data is structured so that extracting insights is possible.
Below are some things you need to know going into the process. Knowing the answers to these questions is the first step of the process of integrating data and establishing what to automate with business intelligence applications:
1. What business questions need to be answered using the data?
2. Where does the data reside, and what is the best way to extract it?
3. What part of the organization will consume that information (i.e., will it be consumed at the enterprise level or specific lines of business)?
4. What format will this data be consumed (e.g., reporting, web solution, mobile solution, etc.)?
Next, you’ll need your team to write scripts that allow you to extract, transform and load the data. Once extracted, the data is moved to a staging area where it is cleaned and structured for the final destination: the data warehouse.
Once you’ve determined which need or challenge you’re working to solve and your data is aggregated into a form (whether automated or not), a flow can be created. This is when you’ll be able to analyze the data, extract insights and show visualizations of advanced analytics to see beyond the obvious.
The final step is to set up the automation of data migration and integration from internal and external sources. This setup is based on the business questions or the necessary data required to run the business efficiently and identifying where the data is located.
Having these best practices in place is the basic principle behind data warehousing design and optimization. It is how to get to that single version of the truth that all can share and use across the enterprise.
Putting It All Together
Through the data warehouse, one enables data modeling, mining and forecasting, as well as chooses which problem to work on first. The data reveals insights that were initially hidden due to the complexity and volume of data that needs to collected and analyzed in real time, or that needs to be collected and reported through mobility.
From there, the scorecards, dashboards, apps and software for visualization become available to test and explore patterns. Patterns can be recognized, and predictive analytics can now answer, “What happens next?” and “How can we make it happen?”
But data itself is one side of the coin.
I believe the other side of the coin boils down to something fundamentally human: Our desire to extend our reach beyond our grasp and, in doing so, go beyond our senses. We are learning to be comfortable in asking a sequence of questions that leads to a prediction more than speculation, and a prescription for an outcome that we might have only imagined.
The bigger picture is to be able to ask new questions and challenge how we are looking at data to truly get an answer to that question of, “What if?”
Originally featured on Forbes.com
Questions? Contact us